|
|
|
|
|
|
|
|
|
|
|
|
|
|
|
|
IC3K is a joint conference composed of three concurrent conferences: KDIR, KEOD and KMIS.
The three conferences are always co-located and held in parallel.
Keynote lectures are plenary sessions and can be attended by all IC3K participants.
Keynote Speakers List:
- Andreas Dengel, German Research Center for Artificial Intelligence (DFKI GmbH), Germany
Title: The Semantic Desktop as a Means for Personal Information Management
- Madjid Fathi, University of Siegen, Germany
Title: Modelling Uncertainties in Advanced Knowledge Management
- David Jensen, University of Massachusetts Amherst, U.S.A.
Title: Knowledge Discovery by Design
- Jan Dietz, Delft University of Technology, The Netherlands
Title:
- Steffen Staab, University of Koblenz-Landau, Germany
Title: Networked Ontologies
- Justin Zhan, Carnegie Mellon University, U.S.A.
Title: Privacy-Preserving Collaborative Data Mining
|
|
|
|
|
|
|
|
|
Keynote Lecture 1 KMIS |
|
|
|
|
|
|
The Semantic Desktop as a Means for Personal Information Management |
|
|
|
|
|
|
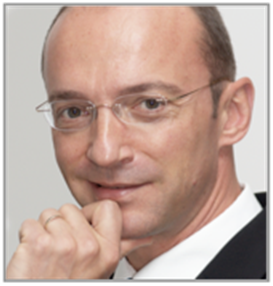 |
|
Andreas Dengel
German Research Center for Artificial Intelligence (DFKI GmbH)
Germany |
|
|
|
|
|
|
Brief Bio
Dr. Andreas Dengel is a member of the Management Board as well as Scientific Director at the German Research Center for Artificial Intelligence (DFKI GmbH) in Kaiserslautern. Since 1993 he is a Professor at the Computer Science Department of the University of Kaiserslautern where he holds the chair “Knowledge-Based Systems”.
He received his Diploma in CS from the University of Kaiserslautern and his PhD from the University of Stuttgart. He also worked at IBM, Siemens, and Xerox Parc. Andreas is member of several international advisory boards, chaired major international conferences, and founded several successful start-up companies. Moreover, he is co-editor of international computer science journals and has written or edited 8 books. He is author of more than 150 peer-reviewed scientific publications and supervised more than 120 PhD and master theses. Andreas is a IAPR Fellow and received prominent international awards. His main scientific emphasis is in the areas of Knowledge Management, Artificial Intelligence, and Document Understanding (for more information, please visit: http://www3.dfki.uni-kl.de/agd/dengel/content/index_eng.html).
Abstract
An inherent problem of the Semantic Web is the provision of a shared vocabulary that is acceptable by many users all of whom having big differences in cultural background, education, role, tasks, and interests. In order to create added value and to convince users of the advantages of the new WWW languages, we focus in a first step on the Semantic Desktop.
A Semantic Desktop is a means to manage all personal information across application borders based on Semantic Web standards. Each resource is identified by a Uniform Resource Identifier (URI) and all data is accessible and queriable as RDF graph. That way, resources from the web can be stored and authored content can be shared with others. Ontologies allow the user to express personal mental models and form the semantic glue interconnecting information and systems. Applications store, read and communicate via ontologies and Semantic Web protocols.
The Semantic Desktop is an enlarged supplement to the user's memory assisting users to file, relate, share, and access all digital information like documents, multimedia, and messages through a Personal Information Model (PIMO). This PIMO is build on ontological knowledge generated through user observations and interactions and may be seen as a formal and semi-formal complement of the user's mental models. Thus it reflects experience and typical user behavior and may be processed by a computer in order to provide proactive and adaptive information support or allows personalizedsemantic search. The Semantic Desktop is built on a middle ware platform allowing to combine information and native applications like the file-system, Mozilla, Thunderbird or MS-Outlook.
After talking about typical problems knowledge workers have to solve today, I will introduce the main concepts, components, and functionalities of the Semantic Desktop. Furthermore, I give examples showing how context-adaptive services on the Semantic Desktop may be used for creating socio-technical collaborative intelligence.
|
|
|
|
|
|
|
|
|
Keynote Lecture 2 KMIS |
|
|
|
|
|
|
Modelling Uncertainties in Advanced Knowledge Management |
|
|
|
|
|
|
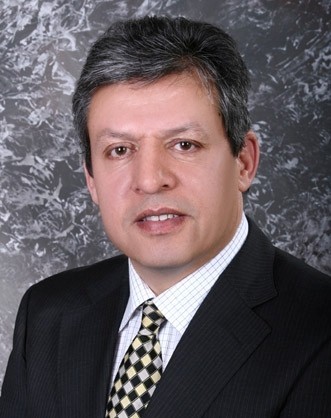 |
|
Madjid Fathi
University of Siegen
Germany |
|
|
|
|
|
|
Brief Bio
Professor Fathi is senior Member of IEEE and the Chair of Institute of Knowledge Based System & Knowledge Management at the University of Siegen in Germany, He is also Director of Center for Knowledge Management and Intelligent System - KMIS at the University of Siegen, Germany.
He has established the International Conference on Knowledge Management for Composites Material, Nano-Technology and Fuel Cell Technology (www.isdt.uni-siegen.de, www.KMCM.Uni-siegen.de) as an annual meeting.
He did his college at the RWTH Aachen, Germany, M. Sc. in Computer Science Univ. of Dortmund, Germany; Ph.D. in Mechanical Eng. Univ. of Dortmund, Habilitation(above Ph.D.) Computer Science Univ. of Ilmenau Germany.
As General Chairman of (IPMM’2001, Vancouver) and Co- Chairman of (IPMM’1999, Hawaii), Inventor and Symposium Chairman of (WAC/ISSCI’96 Montpelier), (WAC/MIP’98 Anchorage), (MIP’2000 Maui), and Co-Chairman of ICCE (‘95-‘00).Associate Editor(AutoSoft Journal, IEEE transaction -SMC, part B). As head of Institute of Knowledge Based System & Knowledge Management (KBS&KM), lead a large of different academic team of researchers and educators which has, thus far, resulted in over 50 thesis. His publication activities at a glance: Number of published works about 180. Books =9 (4 text 2 of them will be finished shortly and 5 Edited,) and book chapters = 2 , 4 Best paper Awards, Conference papers = 150, Journal papers = 20 for a more detailed resume, please consult the web site - www.ws.fb12.uni-siegen.de
Abstract
Handling and managing knowledge is a difficult and complex enterprise. A wide range of advanced technologies have to be invoked in providing assistance for knowledge requirements ranging from acquisition, modeling, (re)using, retrieving, publishing and maintaining of knowledge. Knowledge management originates at least on three roots. At first, suppliers of information technology and academics in this field have developed opportunities of supporting knowledge tasks by knowledge-based systems, artificial intelligence and web-based applications. Secondly, organization and human relations professionals have recognized the need for using the opportunities of an increasingly highly educated work force in modern societies. At last, strategic management has recognized that the optimal use of intellectual capabilities may be the best source for sustaining competitiveness in the global economy.
Knowledge engineers swap ideas, communicate, plan, act, or reason often in situations where facts are unknown and the underlying natural language is uncertain or vague. They do not have access to the complete environment to evaluate each situation. Also conditions are unknown, incomplete or only crudely summarized. Reasoning in realistic domains requires some kind of simplification or adaption to deal with exceptions or to increase the degree of belief in decision making situations. Reasoning under uncertainty is interchangeably associated with the handling of uncertain knowledge. The probability theory has an outstanding position and serves as basis for human’s behavior in decision situations. Uncertainty solutions must solve occurring questions in how to represent uncertain data, how to combine pieces of uncertain data and how to draw inference with uncertain data. Techniques are mainly separated in quantitative and symbolic approaches. Quantitative approaches using certainty factors, fuzzy logic, belief functions, probabilities and possibilities. Symbolic approaches dealing for instance with circumscriptions or default logic.
In this lecture, knowledge representation applications as formal graphical language are examined in detail. Exempli gratia, Bayesian networks are graphical models to represent knowledge under conditions of uncertainty. This network type model the quantitative strength of the connections between variables allowing probabilistic beliefs about them to be updated automatically as new information becomes available. Applications in various fields like mechanical engineering or medicine are exemplified.
|
|
|
|
|
|
|
|
|
Keynote Lecture 3 KDIR |
|
|
|
|
|
|
Knowledge Discovery by Design |
|
|
|
|
|
|
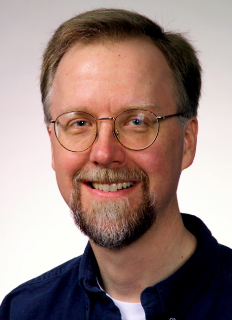 |
|
David Jensen
University of Massachusetts Amherst
U.S.A. |
|
|
|
|
|
|
Brief Bio
David Jensen is Associate Professor of Computer Science and Director of the Knowledge Discovery Laboratory at the University of Massachusetts Amherst. From 1991 to 1995, he served as an analyst with the Office of Technology Assessment, an agency of the United States Congress. He received his doctorate from Washington University in St. Louis in 1992. His research focuses on machine learning and knowledge discovery in complex data sets, with applications to social science, web mining, and fraud detection. He serves on the Executive Committee of the ACM Special Interest Group on Knowledge Discovery and Data Mining and on the program committees of the International Conference on Machine Learning and the International Conference on Knowledge Discovery and Data Mining. He is an associate editor of ACM Transactions on Knowledge Discovery from Data, and he serves on the editorial board of Machine Learning.
Abstract
Traditional work in knowledge discovery focuses on identifying statistical associations. However, increasing numbers of applications require knowledge about cause and effect, rather than mere association. For example, while a decision tree can estimate the probability that a patient will contract a particular disease, it does not provide guidance about how to prevent that disease. Similarly, while associational rules can predict which products will be purchased together, they do not indicate what actions will increase sales of those products. In this talk, I describe some surprising and powerful new opportunities for discovering causal knowledge from observational data. These opportunities exploit recent developments in relational and temporal knowledge discovery. They also draw on a large set of manual techniques for knowledge discovery called quasi-experimental designs. These techniques are widely employed by economists, medical researchers, and social scientists, yet they have never been exploited within knowledge discovery algorithms. Together, these opportunities point to a broad class of algorithms for discovering causal knowledge that can be developed in the next several years.
|
|
|
|
|
|
|
|
|
Keynote Lecture 4 KEOD |
|
|
|
|
|
|
|
|
|
|
|
|
|
 |
|
Jan Dietz
Delft University of Technology
The Netherlands |
|
|
|
|
|
|
Brief Bio
Jan L.G. Dietz is professor in Information Systems Design at Delft University of Technology (The Netherlands). He holds a Master degree in Electrical Engineering and a Doctoral degree in Computer Science, and has practitioned business automation for 10 years. He has published over 200 scientific and professional articles as well as several books. His current research interests are in Enterprise Engineering, Enterprise Architecture and Enterprise Ontology. Jan Dietz is the spiritual father of DEMO (Design & Engineering Methodology for Organizations). He is co-founder and chairman of the DEMO Center of Expertise (www.demo.nl). He is also the leader of the Dutch national research program Extensible Architecture Framework (www.xaf.nl). His current interests concern the development of the emerging field of Enterprise Engineering. To proliferate the notion of Enterprise Engineering he has set up the international CIAO! Network (Cooperation & Interoperability – Architecture & Ontology) (www.ciao.tudelft.nl ).
Abstract
Available soon.
|
|
|
|
|
|
|
|
|
Keynote Lecture 5 KEOD |
|
|
|
|
|
|
Networked Ontologies |
|
|
|
|
|
|
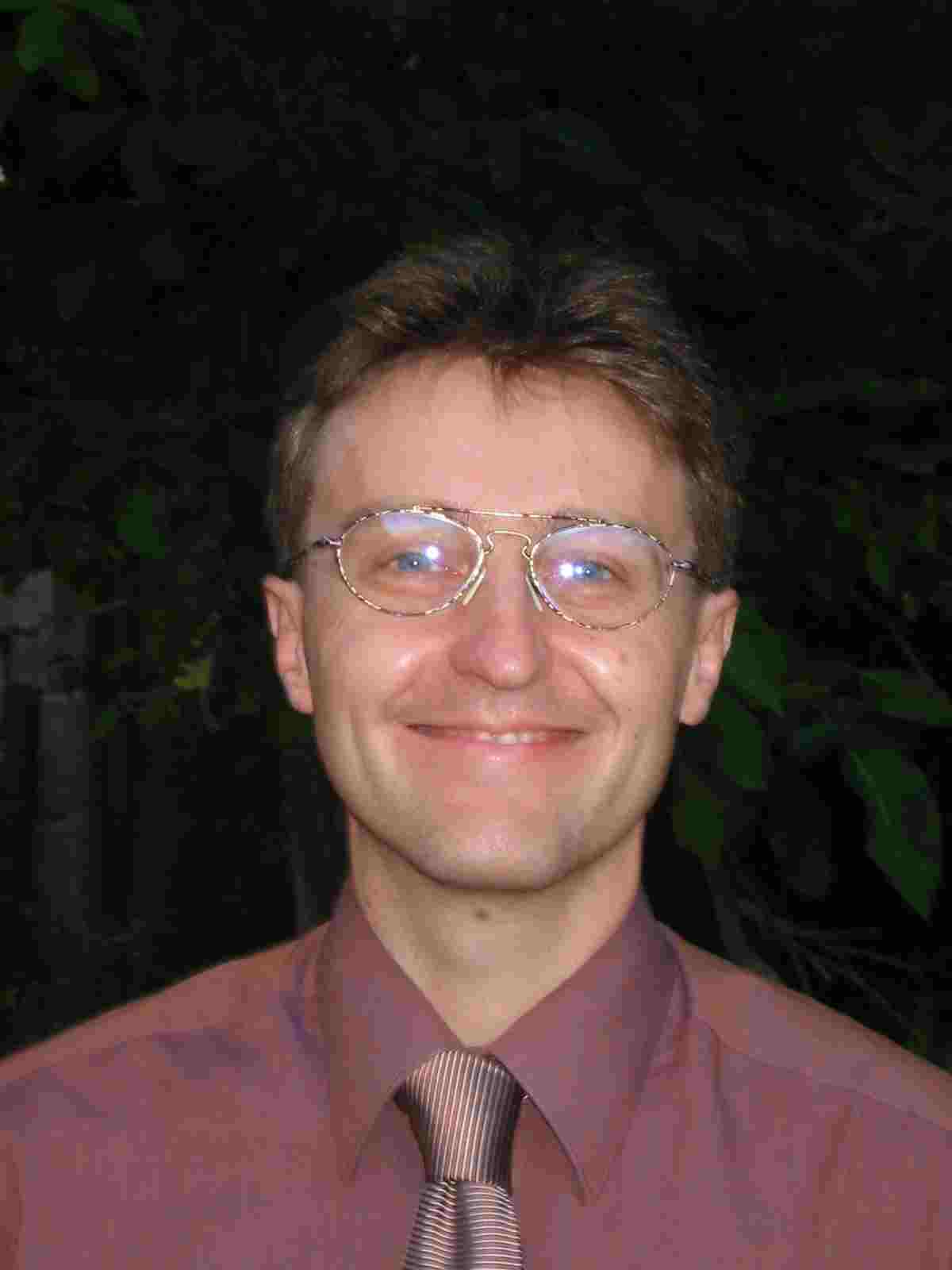 |
|
Steffen Staab
University of Koblenz-Landau
Germany |
|
|
|
|
|
|
Brief Bio
Steffen Staab is professor for databases and information systems at the University of Koblenz-Landau and director of the institute for Web Science and Technologies (WeST) and the institute for Computer Science.
His interests lie in researching core technology for ontologies and semantic web as well as in applied research for exploiting these
technologies for knowledge management, multimedia and software technology. He is Editor-in-Chief of the Journal of Web Semantics and
Department Editor for "Semantic Web" in the IEEE Intelligent Systems.
In 1999, Steffen has co-founded Ontoprise, a worldwide leading company in the field of ontology technologies.
(for more information, see: http://www.uni-koblenz.de/~staab/)
Abstract
In spite of the fact that Ontologies have been put onto the Web for over a decade now, support for true Web Ontologies that are networked with each other is quite limited. The problems stem from limited tool support available for Networked Ontologies as well as from underlying research challenges that have been tackled only recently. In my talk I will present the NeOn toolkit for developing networked ontologies as well as some of our technologies for supporting such networked ontologies, i.e. Networked Graphs and support for provenance and trust when reasoning with Web ontologies.
|
|
|
|
|
|
|
|
|
Keynote Lecture 6 KDIR |
|
|
|
|
|
|
Privacy-Preserving Collaborative Data Mining |
|
|
|
|
|
|
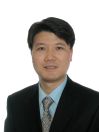 |
|
Justin Zhan
Carnegie Mellon University
U.S.A. |
|
|
|
|
|
|
Brief Bio
Dr. Justin Zhan is a faculty member at Carnegie Mellon University and a research director of Privacy, Security, Social and Service Computing group. His research interests include privacy and security aspect of data mining, privacy and security issues in social networks, privacy-preserving scientific computing, privacy-preserving electronic business, artificial intelligence applied in the information security domain, data mining approaches for privacy management, and security technologies associated with compliance and security intelligence. He has served as a conference chair/committee member for over one-hundred international conferences and an editor/advisory/editorial board member for a dozen of international journals. Recent years, he has published more than one hundred articles in various peer reviewed journals and conferences.
Abstract
Data mining is a process to extract useful knowledge from large amounts of data. To conduct data mining, we often need to collect data from various resources. However, the data are sometimes distributed among and owned by different parties. Privacy concerns may prevent the parties from directly sharing the actual values of data and some types of information about the data. How multiple parties can collaboratively conduct data mining without breaching data privacy presents a grand challenge. Theoretical results from the area of secure multi-party computation show that one may provide secure protocols for any multi-party computation with honest majority. However, the general methods are far from efficient and practical for computing complex functions on inputs consisting of large sets of data. Therefore, to efficiently tackle the problem, formulated as Privacy-Preserving Collaborative Data Mining (PPDM), we need to develop privacy-conscious solutions with adequate efficiency. Our goal is to provide efficient solutions to the problem of data sharing among multiple parties involved in a data mining task, without disclosing the data between the parties. We have developed various privacy-oriented protocols for multiple parties to conduct the desired data mining tasks. We provide efficient solutions to obtain accurate data mining results and minimize private data disclosure. The solutions are distributed, i.e., there is no centralized, trusted party having access to all the data. Instead, we develop secure protocols to exchange the data while preserving the data privacy. In this talk, I will introduce the challenges of PPDM, present solutions, and sketch future directions for this research.
|
|
|
|
|
|
Copyright © INSTICC
Page updated on
25/01/10
|
|